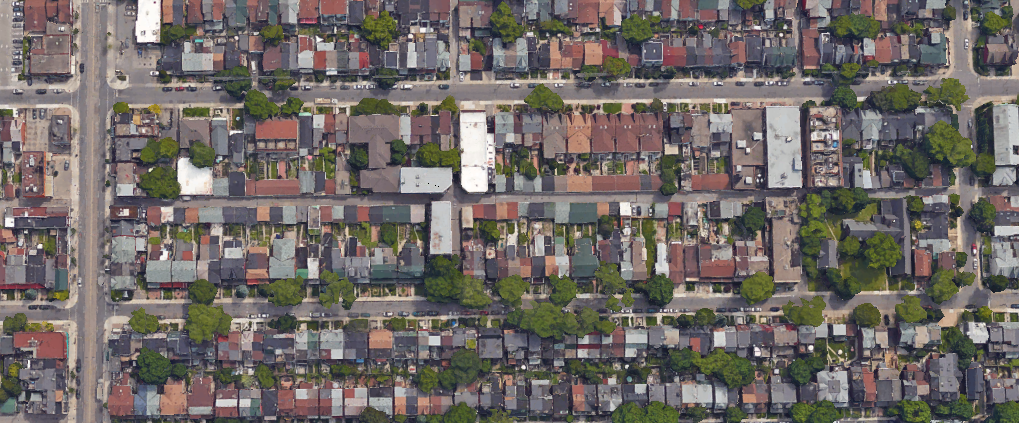
Main Street Data Primer
A Resource for Policymakers, Main Street Stakeholders and Urban Researchers
By Andy Yan, Director of The City Program at Simon Fraser University
The collection and use of data about main streets is essential for understanding the impacts of the COVID-19 pandemic, and for informing the development of strategies and interventions to support economic recovery. COVID-19 has been an accelerator of long-standing trends and changes in retail and services on main streets, amplifying pre-existing weaknesses, and inequalities. Having the right data and analytic approaches are key to addressing these issues.
What problem are you trying to solve for on Main Street? What analysis are you trying to undertake to inform your solution? And what data is available to inform you analysis? These types of questions are critical, as early decisions in shaping your problem definition can have dramatic effects in the amount of resources that a data research project can consume, and the utility that such a project can provide to the community.
This primer was developed as a guide for researchers, analysts, advocates, and advisors navigating through oceans of data sources to inform urban and main street recovery planning. It highlights core data concepts and methodologies, provides tips and tactics for data collection and analysis, and provides a list of data sources that can support data-driven studies.
1. Your Urban Data Research Project: Key Questions to Ask Before You Start
Data projects can be very resource intensive in terms of time, labour, and cost. It is critical to begin with a clearly defined problem and research question that a project is trying to solve. This why question is the core from which subsequent questions – who is the audience; where is the geography; when is the time period – can be asked. Finally, what kinds of data are needed, and how it should to be collected, complete the series of questions to answer in designing a successful data project.
Why? – The Question at The Core of Every Data Research Project
In its simplest form, the “why” is a problem statement that the project is attempting to solve. For example, “we need to understand the economic effects of COVID-19 on Main Street businesses” presents a clear problem statement, framing the project in terms of what the project is attempting to accomplish. Problem statements are not necessarily static, but can change and become more refined over the course of project as more and better data becomes available. Using our example, “economic effects” could be further refined to examine specific characteristics like commercial vacancies or lost revenue. The problem statement can be honed as you progress to answer the questions of who, where, what and how that follow.
Who? – The Audience for Your Data Research Project
The question of “who” is about considering the audience for a data project, which informs the tone and how much content can be communicated in terms of length, style, and design. Will the data be presented in a report that is going to be read by the general public and media, or by policymakers, academic experts and technical staff with specialized knowledge in areas like public and private finance, public policy, or urban development? If there will be a mix of these audiences, you will likely want to limit the technical jargon that should be used. Simple and plain language, as well as strong graphics and visualizations, will dramatically increase the usefulness and communicative value of a data project.
“Simple and plain language as well as strong graphics and visualizations will dramatically increase the usefulness and communicative value of a data project.”
As seen in Figure 1, charts and graphics can often portray information much more effectively than numeric tables and text, conveying trends and patterns much more effectively and quickly for readers. In the case, this chart attempts to convey size of businesses in Quebec and British Columbia based on number of employees, revealing through a simple visual that the vast majority employ less than nine employees.
Figure 1: Data Tables vs Charts
Where? – The Geographic Scale of Your Analysis
Establishing the geographic scale is a fundamental element for a data project. This has always been challenging for urban policy research.
Statistic Canada’s Census geographies are the geographic levels of detail that the federal government and most levels of government organize and provide their datasets based upon. The Census is often freely available at the national, provincial, metropolitan, and municipality (also known as a census subdivision) level. More detailed geographies at a census tract or dissemination area might be available, but may not match local definitions of a non-standardized geography like a neighbourhood or business improvement area (BIA). In these cases, a custom tabulation may incur a cost from the public or private data provider at these geographical levels. Certain public datasets might not be available at a detailed geographic level due to privacy concerns or methodology limitations.
Figure 2: Simplified Hierarchy of Census Geographic Areas
Source: Statistics Canada, Introduction to the geography universe. Catalogue no. 98-301-X, https://www12.statcan.gc.ca/census-recensement/2016/ref/dict/geoint-eng.cfm#moreinfo
Custom geographies like trade areas for specific businesses or business districts can also be useful geographic measures. Buffers of 400 to 800 meters around neighbourhoods or a BIA are often used to define trade areas – the geographic areas that might generate the majority of a main street business’ customers. In practical terms, these distances represent how far a prospective customer can comfortably walk or roll in 15 to 30 minutes, with non-inclement weather conditions. These buffers are also used around transit stations to help set boundaries for “transit oriented” development.
When? – The Baseline and Time Horizon
The timeframe of study is another key element for consideration. Establishing baseline data before and after the declaration of the COVID-19 public health emergency (where possible) provides an important reference to observe the extent of changes that the pandemic has manifested in a neighbourhood.
For example, if your data project is analyzing the impacts of the pandemic on Main Street retail and commercial conditions, understanding when the pandemic began to take effect is a critical consideration. The figure below presents the dates when each province and territory in Canada made their declarations of a public health emergency, which is a good proxy for the beginning of commercial disruption on Main Street.
Figure 3: Public Health Emergency Declarations by Province and Territory
With these dates, there are two ways you could establish a baseline:
Before and after the public health emergency declaration: For example, the two iterations of the Statistics Canada Canadian Survey on Business Conditions have used the reference periods of prior to February 1, 2020 and March 31, 2020 as baselines, with respondents providing before and after responses about the effects that COVID-19 has had on their businesses.
A standardized timeframe: Another approach could be using a benchmark of a time before and after COVID-19 on a standardized timeframe such as one year apart, which can help minimize seasonal economic fluctuations or effects like weather. For example, the one-year timeframe from June 15, 2019 to June 15, 2020 provides a reference that accounts for a period after the public health emergency was declared, but controls for factors like climate seasonality and annual economic season variations.
The challenge of defining “when” is typically dependent on the availability of past data and, in the case of COVID-19 analysis, if a measure can be replicated after the emergency was declared.
What? – The Unit of Measurement and Data Sources You Will Use
The units of measure you choose will obviously relate closely to the kinds of policy and research questions your project focuses on, as well as the audience that might use your research to develop and implement policies. For example, in considering research questions around main street small business and economic conditions, the unit of measure could include businesses or firms (as economic and legal entities), storefronts (the physical space), or owners and workers (individuals).
Within the various economic and social datasets that are available, there are some basic concepts and definitions to consider in terms of organizing and interpreting data for application at the main street scale. Two key concepts are described.
The North American Industrial Classification System
If the unit of measure is the firm, one common way to categorize businesses is the use of the North American Industrial Classification System (NAICS). The system was developed to provide a common classification scheme to organize industries and used to allow for the comparison of firms and industries across various geographic entities like neighbourhoods and cities.
Small and Medium Sized Enterprises, and Independent and Chain Businesses
Where research questions focus more narrowly on small and independent firms as the unit of measure, understanding the definitional nuances is important. With 98 percent of businesses with employees in Canada being defined as “small” (1-99 employees), they are the backbone of most commercial main streets. Yet, this Statistics Canada definition is seen by some as too broad, including businesses that are “too large to be small.”
Bring Back Main Street Memo #6 discusses the Statistics Canada definitions of “small business,” “independent business” and “micro-business,” which are the basis for data collection. As the memo recommends, the “independent business” category is perhaps the most reliable count of small businesses, defined as having one to three outlets operating in the same industry class under the same legal ownership at any time during the survey year. For comparative purposes, a “chain business” is an organization operating four or more outlets in the same industry class under the same legal ownership.
“With 98 percent of businesses being defined as “small” (1-99 employees), they are the backbone of most Main Street commercial enterprises.”
How? – The Methods for Collecting and Using the Data
Data can be collected through a number of methods – each with their own strengths and limitations as you seek a balance between timeliness, representativeness, and cost.
A number of new datasets have been built using various methods by government agencies like Statistics Canada and non-governmental groups like Canadian Federation of Independent Businesses to quickly assess the economic and social effects of COVID-19 on people, businesses and communities. There are also a number of well-established, statistically representative, economic and demographic datasets like the Canadian Census that provide critical context on businesses and the social and economic conditions before the COVID-19 health emergency. Unfortunately, from a timeliness perspective, the latest Census data is from 2016.
This section, about the “how,”, describes the methods that are most commonly being used, especially when conducting research and analysis of “real time” questions related to the COVID-19 pandemic.
Crowdsourcing vs statistical sampling: Some datasets are gathered through mass appeals for responses to web based surveys from certain populations (crowdsourcing) or selecting respondents to ensure answers to surveys can extrapolated to larger populations (statistical sampling). This data can be timely and relatively affordable to gather and administrate, but results may be biased to certain groups that are more likely to respond to the survey. Data that is acquired via statistical sampling may be better representative of a population, but can be expensive to acquire (particularly for specific or smaller populations and communities) and time consuming to process.
Administrative datasets: Tracking functions like business licenses and permits, this type of dataset is gathered by the tabulation and summary of the operations of government administration and regulation. These datasets are dependent on existing government open data policies and portals, which can differ vastly by municipality and may or may not be available to the general public at all. Accessing and interpreting this data will usually require a level of technical acumen, with intermediate and advanced data analysis and visualization.
Hyper-local primary data gathering: For specific neighbourhood scales, primary data gathering where surveyors document vacancies, space sizes, and business mixes storefront by storefront in a neighbourhood can offer insights of detailed hyper-local business conditions. These types of surveys can be very time and resource intensive to conduct but can often be more timely and customizable than administrative datasets.
Mobile location data: Innovations in “Big Data” like credit card transactions, mobile location data, and transportation counts offer the benefit of timeliness and insight. While less prone to human response bias and errors, these datasets are resource intensive to obtain and interpret.
All data methodologies have their respective strengths, weaknesses, and trade-offs. Absolute accuracy and precision may not necessarily be as important or attainable as the usage of datasets that support reasonably reliable findings to support or challenge a direction or decision.
2. Your Urban Research Methods: Tips, Tactics and Considerations
Qualitative and Quantitative Data: The Importance of Narrative
While this data guide largely focuses on quantitative (numbers) data, the power of qualitative (stories and narratives) data should not be underestimated. Narratives help frame numbers by connecting them to the stories and experiences of people and communities. Research suggests that, by the nature of human cognition, stories are more memorable and action driven for most audiences than a table of numbers or data visualization. Data projects can be informed by both quantitative and qualitative analysis, which are not mutually exclusive.
“Narratives help frame numbers by connecting them to the stories and experiences of people and communities.”
Ground-truthing quantitative data through qualitative processes can be used as a means of bringing a community together. Data can be verified and challenged by convening a meeting of community stakeholders and data analysts to explore if the data matches experiences on the ground. In reconnecting data with the communities that generated them, the process of exchanging knowledge in context, nuance, and detail can help inform how to bring main streets back.
Documentation, Sourcing, and Timeliness
The documentation of data sources is the foundation of good data work practice. Given the time required to gather, process, and distribute data, disclosing the date of when a dataset was generated allows readers to understand the timeliness of the data. Data can quickly become stale and is best used to indicate the direction of what is happening as opposed to an exact measure. The documentation of sources is critical in understanding the strengths and limitations of what the data might explain and what it might not.
Representativeness, Bias, and Inclusion
A major concern for any data work is representativeness, bias, and the quality of inclusion. Depending on methods and respondent pools, systemic barriers like limited English or French language proficiency the comfort level of particular populations towards filling surveys, technology access, question phrasing, or respondent pool selection can all undercut the representativeness of a survey. They may accidently (or purposely) exclude entire ethnocultural and demographic groups and produce sizable data gaps for particular populations or geographies in a community. Surveys often struggle with inclusiveness, but there are methods to attempt to improve representativeness.
With the diversity and complexities of urban economies, statistics may best measure the formal economy, but not necessarily the informal economy of a community and those who participate in it. It is advisable to consider whether your data raises concerns of representativeness, bias and inclusion, recognizing the limitations of what your data capture and whom it might exclude.
3. Main Street Business and Demographic Data Sources
There are many existing datasets that allow for analysis of the health, vitality, and conditions of main streets in the context of COVID-19 impacts and recovery planning, and the larger economic and demographic context. While not an exhaustive list, the data sources cited in this primer are largely free and available on the internet, though there may be a charge for more specific custom geographic inquiries by the data provider.
Publicly Available, Largely Free Data Sources
Canadian Business Counts
Example Use: A city would like to get a count of the number of businesses with and without employees by industry.
Source: Statistics Canada’s Business Register which is the central listing of Canadian businesses. They are based on the statistical concept of "location"—that is, each operating location is separately counted, including cases where one business comprises multiple locations. For more details, see https://www23.statcan.gc.ca/imdb/p2SV.pl?Function=getSurvey&SDDS=1105
Website: https://www150.statcan.gc.ca/n1/daily-quotidien/190812/dq190812b-eng.htm
Geography: Nationwide and Province; Custom data queries are available at more detailed geographies
Unit of Measure: Firms by NAICS with employees and without employees
Cost: Free for national and provincial level data, but more detail geographies will require a custom query at a cost.
Limitations: Firms with “no employees” can be difficult to track; there is a spectrum of firms that do not maintain an employee payroll, but may have a workforce which consists of contracted workers, family members or business owners, or simply be “paper firms.”
Municipal Business Licenses, 2019
Example Use: A city would like to get a understand the types and mix of businesses that might be in a Business Improvement Area.
Source: Dependent on the individual municipal open data policies
Sample Websites: https://opendata.vancouver.ca/explore/dataset/business-licences/information/?disjunctive.status&disjunctive.businesssubtype | https://data.surrey.ca/dataset/business-licences/
Geography: Customizable to the Individual location of the business license
Unit of Measure: Individual Business Licenses by regulatory category
Cost: Data is typically free, but the expense will be incurred in creating the analysis, visualization, and mapping of the data.
Limitations: Multiple licenses may be held by a single business and not all businesses may be required to have a business license and miscategorizations can occur.
Retail/Commercial Vacancy Information
Example Use: A Business Improvement Area would like to learn about vacancies in its neighbourhood and its citywide context.
Source: Various private providers but Cushman Wakefield, Colliers International, and CBRE offers examples of what is available.
Website: https://www.cushmanwakefield.com/en/canada/insights/canada-marketbeats
https://www.collierscanada.com/en-CA
https://www.cbre.ca/en/research-and-reports
Geography: Citywide and some neighbourhoods
Unit of Measure: Storefront Vacancies
Cost: Free
Limitations: Citywide geographies may not offer the desired levels of geographic details.
Canadian Survey on Business Conditions: Impact of COVID-19 on businesses in Canada, April and July 2020
Example Use: A survey of business conditions before and after COVID-19 public health emergency declarations
Source: Statistics Canada and the Canadian Chamber of Commerce
Website: https://www150.statcan.gc.ca/n1/daily-quotidien/200429/dq200429a-cansim-eng.htm (March 2020) https://www150.statcan.gc.ca/n1/daily-quotidien/200714/dq200714a-cansim-eng.htm (May 2020)
Geography: Nationwide and Province with selected Metropolitan Areas and Municipalities (sample size sensitive)
Unit of Measure: Firms by NAICS with additional information on Number of Employees firm size; Ownership status; majority ownership status on woman, First Nations, visible minorities, immigrant to Canada, person with a disability; and business activity.
Cost: Free
Limitations: Crowdsourced Internet Survey with representative sampling limitations
Investigating the Impact of COVID-19 on Independent Business
Example Use: A general survey of businesses and the impact of COVID-19 on business conditions and recovery status.
Source: Canadian Federation of Independent Businesses
Geography: Nationwide with provincial breakdowns
Unit of Measure: Individual businesses
Cost: Free
Limitations: The data is limited to a crowdsourced web-based survey and respondents who are members of the Canadian Federation of Independent Businesses.
2016 Canadian Census
Example Use: A Business Improvement Area would like to know the demographic, economic and social context of the City
Source: Statistics Canada
Website: https://www12.statcan.gc.ca/census-recensement/2016/dp-pd/prof/index.cfm?Lang=E
Geography: Nationwide, Province, Region, and Municipalities
Unit of Measure: Households, Families, and Individuals
Cost: Free
Limitations: For detailed and custom geographies like neighbourhoods, a high level of census data knowledge is required. Datasets reflect data as from the Census 2016.
Private, Paid Data Sources
For more timely commercial/retail vacancies and demographics data, users may want to consider the following private sources. The major limitation for these kinds of datasets tend to be cost as detailed information requires joining a subscription service or engaging a firm for consultation services. Moreover, for small markets and communities, this data may not be available.
Commercial and Retail Vacancy
Example Use: A Business Improvement Area would like to acquire very geographically specific data on commercial and retail vacancies.
Source: Costar
Website: https://www.costar.com/
Geography: Customizable
Unit of Measure: Storefronts
Cost: Subscription Based
Limitations: Proprietary datasets and sources which may have limited neighbourhood coverage depending on area and community.
Demographics
Example Use: A Business Improvement Area would like to obtained detail and more timely demographic data and profiles for a neighbourhood.
Source: Environics Analytics
Website: https://environicsanalytics.com/en-ca/data
Geography: Customizable
Unit of Measure: Individuals
Cost: Subscription Based
Limitations: The data is generated through proprietary methodologies and public and private data sources, but is provides a more time demographic portrait of a community.